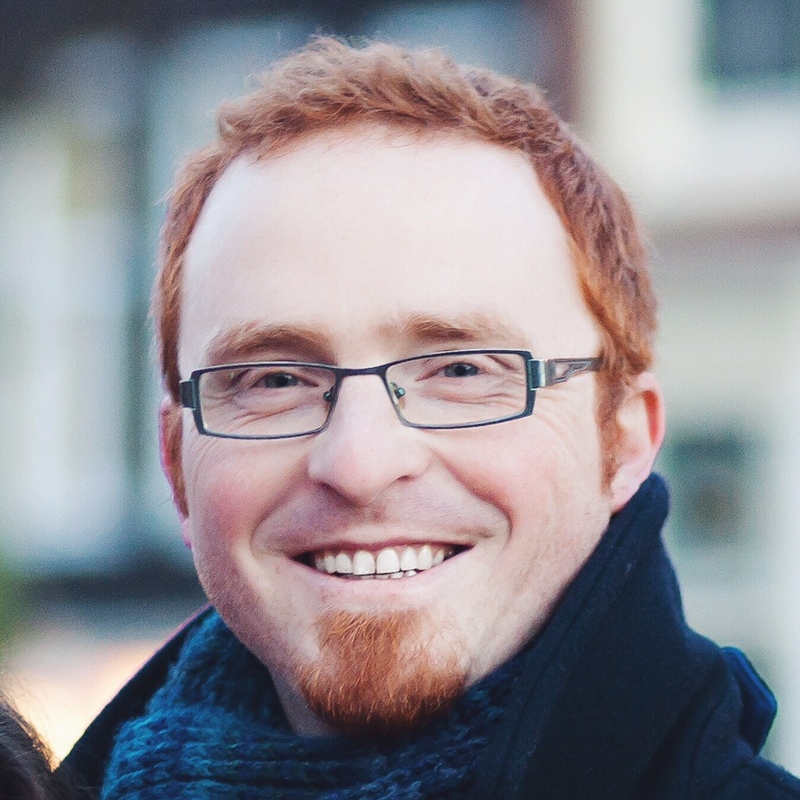
Dr. Raf Van de Plas
Raf Van de Plas is Associate Professor at the Delft University of Technology (TU Delft) in the Netherlands, within the Delft Center for Systems and Control. He works on the mathematical analysis of spectral imaging systems (e.g. imaging mass spectrometry), signal processing and machine learning for molecular imaging modalities (e.g. microscopy), and data-driven multi-modal fusion between imaging technologies. He is furthermore appointed Adjunct Assistant Professor at the Vanderbilt School of Medicine at Vanderbilt University in Nashville, TN in the United States.
Dr. Van de Plas obtained his Ph.D. in Engineering Sciences at the KU Leuven (Belgium) on the topic of Multivariate Datamining Strategies for Imaging Mass Spectrometry in 2010. He holds a degree in Industrial Engineering (Groep T, 2002) and an Advanced Master in Artificial Intelligence (KU Leuven, 2003). Before starting at the TU Delft in 2014, he held a post-doc and later a faculty position at the Mass Spectrometry Research Center (MSRC) at Vanderbilt University in Nashville, TN (United States).
Dr. Van de Plas' research focuses on the interface between three different fields: (i) mathematical engineering and machine learning; (ii) analytical chemistry and instrumentation physics; and (iii) life sciences and medicine. His research is concentrated at the cross-section between these areas, with a focus towards deepening the understanding of in-tissue bio-molecular processes and patho-mechanisms.
Current members
Dr. Lukasz Migas
Post-Doc, Feb 2019 - present
Lukasz joined the lab after finishing his PhD at The University of Manchester where he was supervised by Prof. Perdita Barran. During his PhD, his research focused on studying biomolecules using native mass spectrometry and ion mobility-mass spectrometry.
Since joining TU Delft, he has been working on developing framework to enable analysis of ion mobility-imaging mass spectrometry (IM-IMS) data. His research focuses on developing efficient file format for IM-IMS data as well as various analysis pipelines that enable high-performance signal alignment and automated feature detection in multiple dimensions.
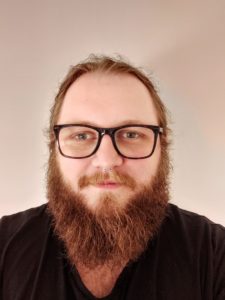
Leonoor Tideman
PhD candidate, Oct 2019 - present
Leonoor started her PhD at the Van de Plas Lab after having obtained her MSc in Systems and Control from the Delft University of Technology. She is a machine learning researcher with a background in mechanical and control engineering. She has expertise in signal processing, statistics, machine learning, deep learning, optimization and hyperspectral imaging. Her research focuses on the analysis of imaging mass spectrometry data using supervised machine learning algorithms, and the question of machine learning model interpretability.
Roger Moens
Master student, 2017 | PhD candidate, Oct 2019 - present
Roger joined the Van de Plas Lab in 2017 performing his BSc thesis on “Detecting Biologically Relevant Patterns in Imaging Mass Spectrometry Signals”. He is currently working towards a double MSc degree in Systems and Control at the Delft Center for Systems and Control and Aerospace Engineering at the Faculty of Aerospace Engineering. His current research focuses on robust factorization methods for spectral imaging with applications in astronomical spectroscopy and imaging mass spectrometry. Fields of interest: numerical linear algebra, convex optimization, and extreme scaling.
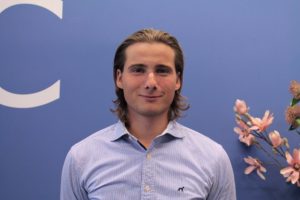
Former members
Dr. Nico Verbeeck
Post-Doc, Sep 2015-Jun 2017 | now at Aspect Analytics
Nico Verbeeck joined the Van de Plas Lab as a post-doctoral researcher. He obtained his PhD at the STADIUS center for Dynamical Systems, Signal Processing and Data Analytics, KU Leuven, Belgium on the topic of "Data mining of imaging mass spectrometry data for biomedical applications" in 2014. He also holds a Master in Engineering in Nanotechnology (KU Leuven, 2008) and a Master in Artificial Intelligence (KU Leuven, 2009).
Dr. Verbeeck works on the development of new methods for automated interpretation and exploration of imaging mass spectrometry (IMS) data, by combining IMS data with external information sources such as anatomical atlases, in order to leverage data analysis and enable guided exploration of these large datasets. Further research topics include dimensionality reduction and feature selection in IMS data through wavelet analysis and multivariate decomposition techniques for comparison of multiple IMS datasets.
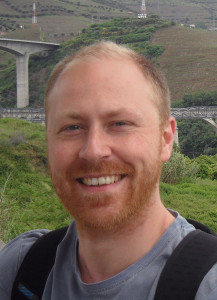